Gender Trouble
4 mistakes you can make with gender as a variable
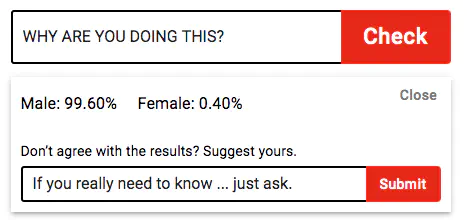
If you’ve spent anytime on tech twitter in the last 48 hours, you might have noticed a lot of people dunking on a product called Genderify, an api for guessing the gender of clients based on their names or usernames.
The idea of a paid product to predict gender is pretty absurd. The cheapest and most accurate way to get gender data for individuals is to just ask!
However, just asking can be more complicated than it appears on the surface. Here’s a quick (and incomprehensive) list of common mistakes you can make when working with gender (or sex) as a variable.
1. Conflating sex and gender.
Sex corresponds to reproductive biology and is mostly inferred by a child’s anatomy at birth. Gender corresponds to social role and internal identity. Sex and gender are equivalent for many people, but they aren’t always. Cisgender people identify with the gender they were assigned at birth, while transgender people do not.
Unless your research has a reason to be concerned with biology, always ask for gender.
2. Using gender as a proxy for other variables.
Does your analysis or model actually care about gender or does it actually care about a gendered behavior or trait?
If your model shows that women are more likely than men to buy your laundry detergent, it’s likely not just because they are women, but that gender is operating as proxy for another variable that’s associated with gender such as being responsible for the household laundry. You’ve also potentially lost valuable decision-making information about the relationship between specific behaviors and other variables.
It’s not always possible to obtain information about specific behaviors or traits, but modeling and making decisions based on gendered associations rather than the traits themselves can reinforce stereotypes and biases.
3. Accidentally introducing gender as a variable.
In the same way gender can act as a proxy for other variables, other variables can act as a proxy for gender.
Apple famously created an algorithm that discriminated on the basis of gender, even though gender wasn’t a variable in the model.
Using variables strongly associated with gender can embed gender as a feature in your model even if you don’t intend to include it.
4. Using bad gender categories in your data collection.
I see this mistake a lot in attempts to be inclusive of transgender people. If you are asking people to self-report gender, don’t include “male”, and “female to male” as options in the same field. While there are definitely differences in experience between cisgender men and transgender men, this language implies that trangender men are not male.
In addition to not being a good classification system, in most scenarios, it’s not necessary to know if a person is transgender and invasive to ask.
It is also important to allow people to identify as non-binary or to not list a gender identity in your data collection as well.
Cover photo from a tweet by Sasha Costanza-Choc